Why Every Product Manager Building in AI Needs to Start Thinking About LLM User Analytics
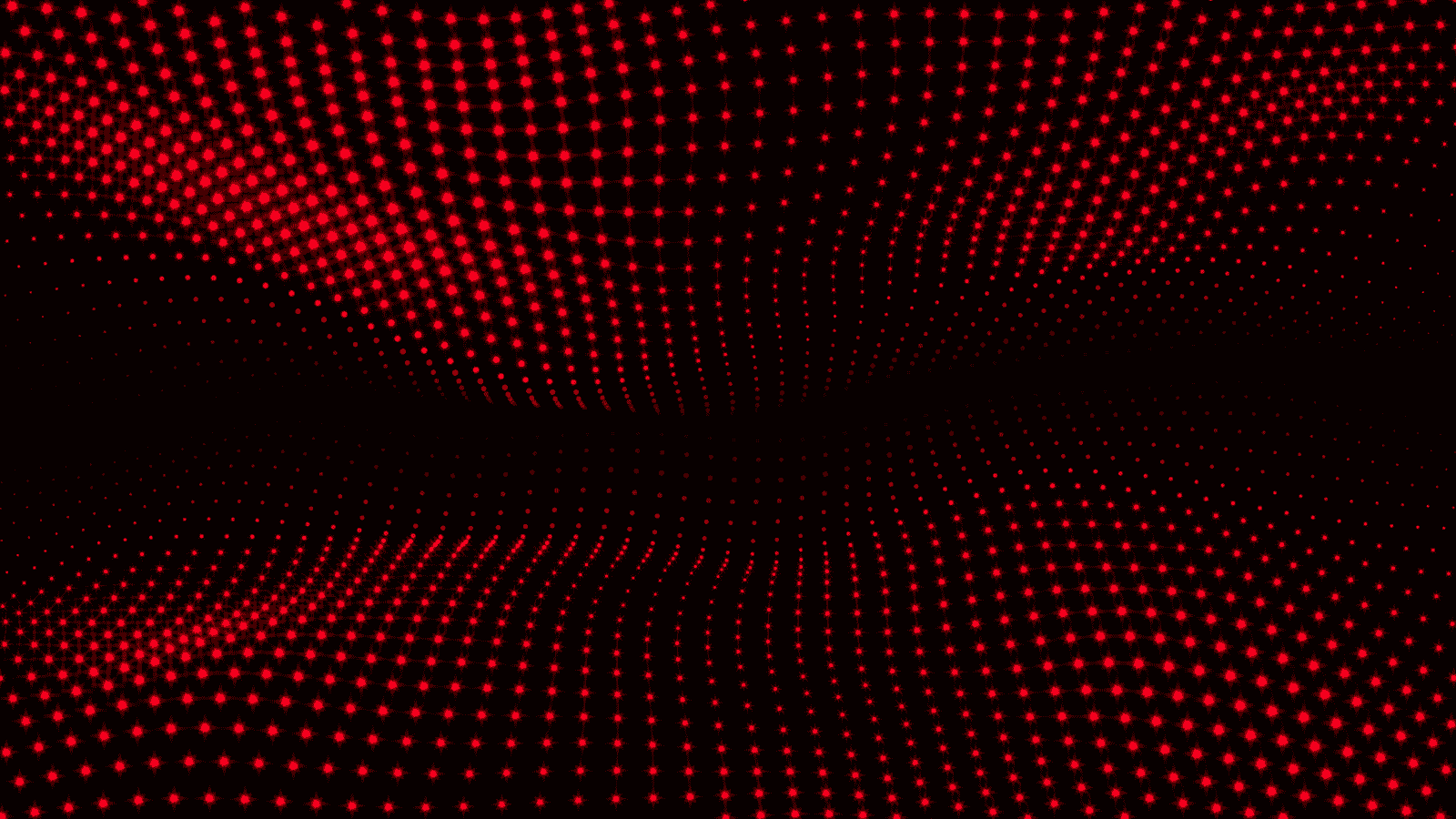
AI-driven products are revolutionising user experiences, and at the heart of this transformation lies conversational interfaces powered by LLMs. As a product manager, you’re tasked with steering these innovative tools to success. But here’s the catch: the traditional metrics and frameworks you’ve relied on for web and mobile analytics are not comprehensive enough for this new frontier.
If you’re building in AI and not yet thinking about LLM user analytics, you’re missing a vital piece of the puzzle. This emerging type of analytics is the key to unlocking user insights, improving your product, and ensuring its success in an increasingly competitive space. Much like web analytics defined the success of the early internet era, LLM user analytics is poised to do the same for conversational AI. Let’s dive into why it matters.
Why LLM User Analytics Matters for Product Managers
1. Driving Product Improvements Through Data
Good product management thrives on data-driven decision-making, and LLM user analytics provides an additional layer of insights that traditional tools do not cover. New tools go beyond tracking engagement or retention, offering a granular look at conversation quality, user sentiment, and intent. Imagine understanding not just how users interact with your AI but also why they’re engaging and where the experience falls short.
For example, is your AI correctly interpreting user intent? Are conversations leading to successful outcomes? These insights are often hidden within the nuances of the conversational flow and sentiment, requiring a different type of analysis. Extracting these insights allows you to refine flows, optimise prompts, and make iterative improvements that align with user needs.
2. Introducing the ABCC Metrics Framework
Traditionally, product managers relied on ABC metrics—Acquisition, Behaviour, and Conversion—to measure the success of their products. While Acquisition metrics (how users are acquired and where they come from) remain relevant in the conversational AI paradigm, Behaviour and Conversion require a significant shift.
- Behaviour (B): Simple click and engagement metrics are no longer enough. Much of the insight into how users engage with conversational AI is hidden within the conversational flow, including sentiment, tone, and intent.
- Conversation (C): In this new paradigm, conversations themselves become critical. Tracking what happens within user dialogues—such as key topics, unresolved queries, and repeated questions—offers a deeper understanding of how effectively the AI meets user needs.
- Conversion (C): Unlike traditional interfaces where conversion might be tracked via a CTA click, in conversational AI, conversions are often embedded within the conversation itself.
Adapting the ABC framework to ABCC highlights how LLM user analytics is essential for uncovering user intent, enabling real-time optimisation, and building more intuitive conversational flows.
Consider a future where an LLM-powered customer support assistant for an e-commerce brand doesn’t just answer product questions but actively assists users in completing purchases directly within the chat. To enable this, product managers need to analyse user dialogues using LLM user analytics tools to identify signals of purchase intent. They can then refine the AI to act on these signals, delivering a seamless, proactive user experience.
3. Balancing Risk and Value Creation
AI product managers operate in a unique space where managing risk is just as critical as delivering value. One misstep—an inaccurate response, a privacy breach, or an inappropriate output—can jeopardise trust and damage your brand. At the same time, overly restrictive guardrails can stifle the AI’s potential to delight and engage users.
LLM user analytics helps you navigate this balance by providing metrics that address both sides of the coin. For instance, monitoring accuracy and safety metrics protects users while also identifying areas where the AI could better meet their expectations.
4. Proving ROI to Stakeholders
As AI investments grow, so does the pressure to demonstrate their return on investment. Product managers need robust analytics to build a compelling ROI narrative. By showcasing how conversational AI improves user satisfaction, reduces friction, or boosts operational efficiency, you can build a case for continued investment. LLM user analytics equips you with the data to answer the tough questions from CEOs and CFOs.
Core Capabilities That Product Managers Need from LLM User Analytics
1. Conversation Quality Metrics
A great AI product starts with strong conversational quality. Metrics like turn completion rates, success rates, and sentiment scores highlight where users are dropping off or feeling frustrated, offering insight into bottlenecks. Advanced analytics such as intent recognition and topic modeling go a step further, identifying patterns in user needs and intent to refine interactions and deliver better outcomes.
2. Real-Time Feedback and Iteration
Iterative improvement is key. Regular analysis of conversation logs, flagged interactions, and sentiment trends ensures that product managers can make data-driven updates to improve flows, prompts, and overall user satisfaction. The goal is agility, not immediacy—steady refinements that align the AI with user expectations.
3. Balancing Guardrail Monitoring with Value Identification
LLM user analytics doesn’t just track what users are doing; it also monitors how the AI is behaving. Tools that enable topic analysis are particularly valuable here. They can identify sensitive topics that require additional review to ensure guardrails are working, while simultaneously uncovering areas where users are seeking information or functionality that the AI cannot yet address.
Practical Steps to Get Started with LLM User Analytics
1. Define Clear Objectives
Start by identifying specific goals for your LLM user analytics efforts. Are you aiming to reduce churn, improve conversational success rates, or strengthen your guardrails? Your objectives will guide the tools and processes you need.
2. Select the Right Tools
Choose tools that align with your needs. Nebuly, for example, excels in analysing user intent and sentiment, while other platforms may focus on guardrail metrics or real-time performance monitoring. Deciding between building in-house capabilities or leveraging these established solutions is key to scaling efficiently.
3. Establish a Feedback Loop
Analytics without action is just noise. Ensure you have processes in place to analyse data, identify opportunities for improvement, and implement changes. By iterating on insights, you create a feedback loop that continuously optimises your AI’s performance, keeping it aligned with both business objectives and user needs.
Final Thoughts: Navigating the New Era of Product Management
The age of conversational AI demands a new playbook for product managers. LLM user analytics is the compass that helps you navigate this evolving landscape, offering the insights needed to improve user satisfaction while safeguarding your brand.
If you’re exploring this space and need guidance, I’m here to help. Send an email to mail@unmuted.ai or find me on LinkedIn to discuss how LLM user analytics can become a core pillar of your product strategy.
Let’s keep the conversation going—unmute your AI and unlock its full potential.