How Our Definition and Understanding of User Sentiment Has to Change in the Age of Conversational AI
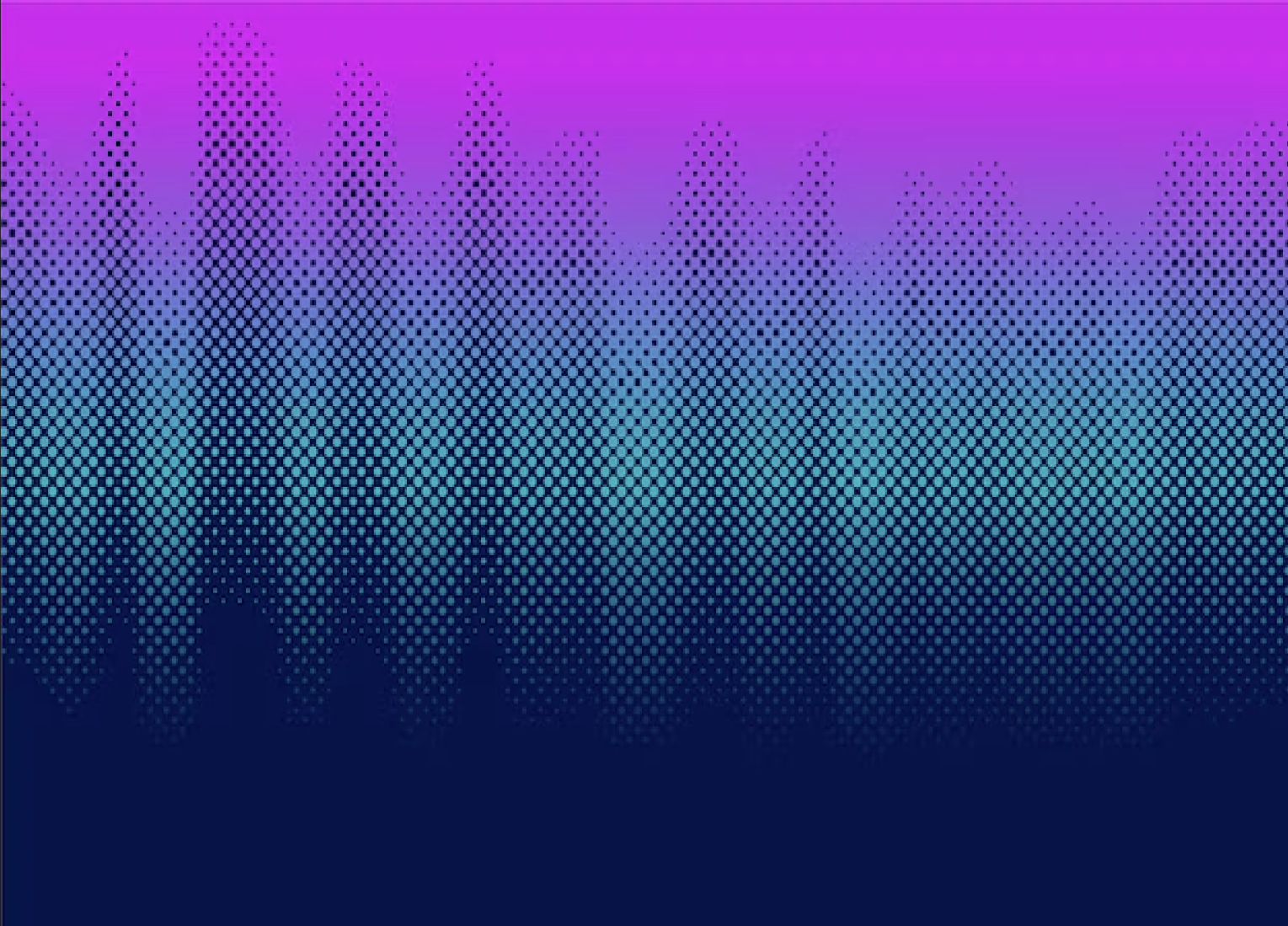
In the world of web analytics, sentiment analysis has always been a useful tool forunderstanding how users feel about your brand - whether it's positive, negative, orsomewhere in between. But here’s the thing: sentiment in the context of data derived from reviews or social media posts is somewhat static and mostly analysed async. With the rise ofconversational AI, we’re entering an entirely new phase of sentiment analysis - one that requires a deeper, real-time understanding of user emotions as they interact with AI-driven systems.
This evolution is not just about tracking how users feel about your brand at a high level; it’s about understanding their moment-by-moment intent, emotional state and level of satisfaction/frustration as they engage in a conversation with AI. If you decide to provide users with a conversational AI interface, it’s time to unmute (see what I did there) these interactions and capture the full spectrum of user sentiment.
1. Traditional Sentiment Analysis vs. Conversation-Based Sentiment in LLMs
Traditional Sentiment Analysis vs. Conversation-Based Sentiment in LLMs
Sentiment analysis of user interactions on the web and social media has traditionally been pretty straightforward - usually focusing on positive, negative, or neutral sentiments derived from static text, such as written reviews or social media posts. While this method has servedits purpose, it falls short in today’s era of conversational AI.
Unlike static data, conversational AI unfolds dynamically. User sentiment can shift throughout the interaction, meaning businesses need a more sophisticated approach. Inconversational interfaces, sentiment isn’t just an indirect measure of brand perception - it’s a direct indicator of the quality of interaction between AI and the user. Conversations evolve,emotions change, and subtle cues like tone and pacing become crucial for assessing the success of these interactions.
This shift calls for new methods of monitoring conversations and tapping into the rich insights that come from real-time, in-depth conversations between users and AI.
2. Challenges: Detecting Nuances Like Sarcasm and Complex Emotional States
Now, here’s where it gets tricky: conversational AI brings a host of new challenges to the table. One of the biggest hurdles is detecting the emotional nuances that arise during real-time interactions. Think about it - users don’t always express themselves plainly. They might throw in sarcasm, humour, or subtle frustration, which are harder for traditional models to pick up.
There are two major challenges here. First, emotions like sarcasm or confusion can easily slip through the cracks of basic sentiment analysis tools. Second, because users have much higher expectations when interacting with AI, they’re more likely to express both positive and negative emotions directly during the interaction. This means businesses need to be equipped to gauge real-time sentiment to ensure their AI is meeting user expectations.
To solve this, LLM-based sentiment analysis relies on more advanced linguistic models that can recognize and interpret these emotional complexities, ensuring that businesses can provide better responses based on real emotional understanding.
3. How LLMs Enable a Deeper Understanding of User Sentiment (Beyond Positive/Negative)
With advanced linguistic models, LLMs are moving sentiment analysis beyond the basic “positive” or “negative” categories. Tools like Context AI and Nebuly have started providing /LLM sentiment capabilities allowing to capture signals such as intent, sentiment and abroader emotional spectrum.
While these tools are a good start, there’s still work to be done. Right now, they providesome capabilities, but the real challenge lies in acting on these insights in real-time. Fully detecting the subtle signals hidden in a user’s query or response, and then feeding that information back to the LLM to adjust the immediate response and/or the conversation flow on the spot, remains an area where more innovation is needed.
Ultimately, tools like these will need to evolve further to fully unmute (3rd time, I’m on a roll) \the potential of conversational AI. The ability to detect rising frustration, for example, andimmediately adjust the AI’s response or escalate the issue to a human agent, will be key tocreating more personalised, responsive interactions. As sentiment tracking dashboardsbecome essential for businesses aiming to improve user satisfaction, the future lies infine-tuning these insights to truly optimise the conversational experience in real-time.
4. Case Study: How Evolving Sentiment Analysis Improves Customer Experiences
Let’s look at an example from the e-commerce world. Imagine a customer service chatbotinteracting with a user who’s trying to make a purchase but gets stuck. Traditionally, the business wouldn’t know if the user was frustrated until they had analysed the transcriptpost-hoc, or until the customer had left a negative review. But with coupling an LLM-drivenconversational customer support chatbot with advanced sentiment analysis, businesses candetect frustration as it happens - say, through repeated queries or escalated tone - and adjust the conversational flow or redirect the user to a human agent before the experiencegoes downhill. That can make the difference between a purchase and a churned customer.At scale, it can make the difference between a successful and a struggling brand.
5. Conclusion: Unmuting Your AI for the New Age of Sentiment Analysis
The world of sentiment analysis is evolving. It’s no longer just about tracking static emotions- it’s about capturing the real-time, nuanced emotional journey of your users as they engagewith conversational AI. By leveraging the power of LLMs and advanced sentiment analysis tools, businesses will be able to truly unmute their AI, unlocking deeper insights that go farbeyond the traditional sentiment metrics of the past.
At the end of the day, understanding user sentiment is key, but what truly sets businessesapart is how they act on those insights. As we’ve learned from web analytics, sentiment isjust the beginning - what matters is how you use that data to continuously optimise your AI’sperformance, creating personalised, real-time experiences that keep users engaged.